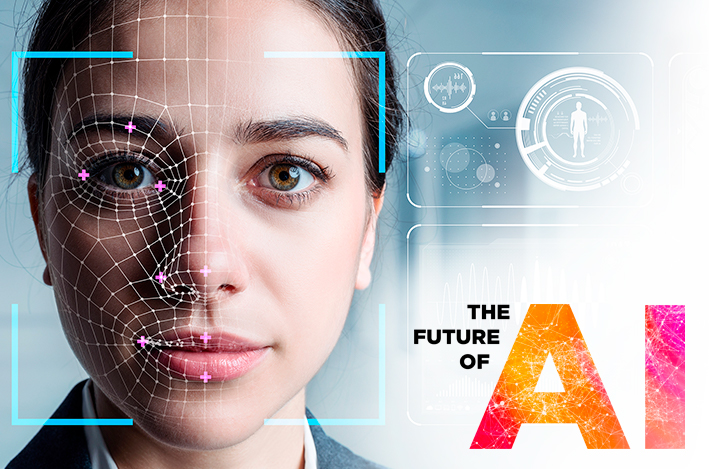
The images that popular media and Hollywood movies evoke about artificial intelligence (AI) differ vastly from reality. Science fiction movies tell stories of generalized AI that is more flexible, human-like and can apply understanding from multiple sources, which inevitably leads to them threatening the human race.
In reality, machines are nowhere near having such generalized capabilities, although that hasn’t stopped prominent figures like Elon Musk stirring public concern about AI’s threat to humanity.
However, the closest concept we currently have to this may be a machine learning (ML) technique called reinforcement technology.
Reinforcement Learning Explained
Reinforcement learning is the generalized AI concept that may have led AI doomsayers to their conclusions. It’s a form of machine learning that rewards positive or desired behavior and punishes negative or undesired behavior. A reinforcement learning agent experiments and learns from its mistakes, then rectifies its actions. The agent gradually learns to take and increase actions or optimal behaviors that result in the maximum reward.
Reinforcement learning doesn’t involve telling a machine whether there’s a particular objective or if it’s achieving it or not. Instead, it’s simply advised whether it’s getting better or worse at specific accomplishments.
Applications of Reinforcement Learning
One of the best-known applications of reinforcement learning is DeepMind’s AlphaGo machine, which used the concept to understand and defeat the world champion of board game Go in 2016. It has also been used by OpenAI to enable a robotic arm to solve the Rubik’s Cube, perform predictive maintenance and solve tax equality and policy issues.
A significant area for reinforcement learning applications is autonomous driving systems, which need to perform multiple tasks in uncertain environments. Reinforcement learning enables these systems to plan vehicle paths and execute motion prediction, such as the movement of pedestrians and other vehicles.
What to Expect from Reinforcement Learning in the Short and Long Term
Despite these advances, we are still some way away from machines having the required level of cognitive capacity to outsmart humans. Reinforcement learning is already widely used in industries like gaming, finance, inventory management and robotics. As a result, it has the potential to be a game-changing technology for the AI space.
In 2018, Facebook announced it was launching a new AI service, Facebook M, that would allow people to go onto Messenger and chat with the AI about anything. In reality, it started with human responses, which Facebook hoped its AI would learn from, but it didn’t work out because it was too difficult. Looking to the future, we can use communication theory to understand human conversations in unconstrained domains.
Reinforcement learning also has a role to play in enhancing human experiences of entertainment. For example, its free-form environments could enable movie and video game makers to create storylines that divert from traditional, fixed dialogues.
Elon Musk’s Stance on AI
Elon Musk, the founder of Tesla and SpaceX, has repeatedly voiced his apprehension about the potential of AI. At the World AI Conference in 2019, he expressed his concerns about the lack of accountability and caution from researchers. This included his claims that people were underestimating the capabilities of AI and that advancements in the technology could lead to it taking over from humans.
Musk has also stated on several occasions that he sees AI as humanity’s “biggest existential threat” and used hyperbole to claim it’s likely we’re all living inside a computer simulation. He also claimed he invested in Google’s DeepMind technology to address his fears of Terminator-like situations being caused by ongoing AI research.
What Uniphore Is Doing for AI
Conversational AI is reliant on advances in a generalized intelligence capacity. Traditionally, our focus has been on natural language processing and language understanding. We’ve made significant moves to identify customer intent on phone calls and understand the type of language people use.
But our vision of the future of AI is primarily built around enhancing the understanding of context and intent. Trying to get machines to understand language is the most challenging problem in the whole AI space. Even the smartest linguists don’t have a clear understanding of why language works the way it does. Therefore, writing down all the rules to help a machine effectively and naturally converse with humans remains complicated and a long way away.
We’re looking to further improve language understanding through multi-modal understanding, which includes combining speech and vision. For example, we recently acquired Emotion Research Labs, which adds video-focused AI capability to our analysis and understanding of real-time voice conversations.
We’ve also established global partnerships that are helping us to develop the next generation of AI, automation and machine learning technologies. For example, the Idiap Research Institute in Switzerland enables us to push the boundaries of what we’ve done so far and the Indian Institute of Technology Madras (IITM) allows us to take a more high-risk research approach.
These collaborations and innovations are helping us work toward developing a computer system that truly understands the process of human communication.
The Exciting Future of AI
Contrary to what popular media and Elon Musk may tell us, the reality of AI’s future is most likely to augment and enhance human behavior. Advances in machines’ understanding of human communication will not lead to them overtaking us or leading to our downfall. Concepts like reinforcement learning will improve conversations and engagement, which will lead to broader business benefits like better customer service, customer automation and sales effectiveness.
Discover how Uniphore can help your organization better interact with customers and increase sales by downloading our Future of AI for Contact Centers ebook.